Part of the Deep Dive: AI Webinar Series
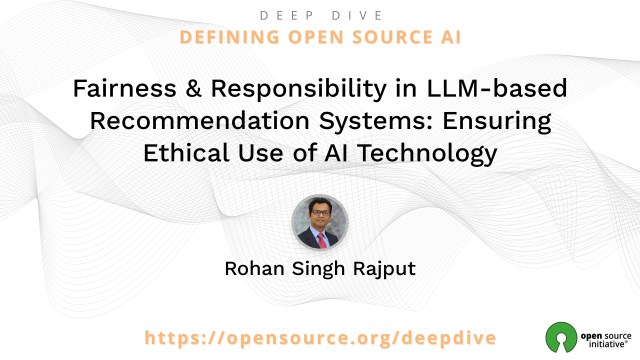
The advent of Large Language Models (LLMs) has opened a new chapter in recommendation systems, enhancing their efficacy and personalization. However, as these AI systems grow in complexity and influence, issues of fairness and responsibility become paramount.This session addresses these crucial aspects, providing an in-depth exploration of ethical concerns in LLM-based recommendation systems, including algorithmic bias, transparency, privacy, and accountability. We’ll delve into strategies for mitigating bias, ensuring data privacy, and promoting responsible AI usage.Through case studies, we’ll examine real-world implications of unfair or irresponsible AI practices, along with successful instances of ethical AI implementations. Finally, we’ll discuss ongoing research and emerging trends in the field of ethical AI.Ideal for AI practitioners, data scientists, and ethicists, this session aims to equip attendees with the knowledge to implement fair and responsible practices in LLM-based recommendation systems.
Webinar Summary
In this webinar hosted by the Open Source Initiative as a part of the “Deep Dive: Defining Open Source AI” series, Rohan Rajput discusses the intersection of fairness and responsibility in Language Model (LM)-based recommendation systems. He begins by introducing LM as powerful language models trained on vast textual data, highlighting their ability to generate responses based on user prompts. Recommendation systems, such as those used by Amazon and Netflix, are then introduced as a domain within Information Retrieval Systems. The main focus of the presentation is on LM-based recommendation systems, categorized into prediction and generation tasks. Rajput emphasizes the ethical challenges these systems face, particularly in domains like education, criminology, finance, and health, due to the potential replication of biases present in training data. He discusses fairness and bias mitigation strategies, including robust data processing, algorithmic fairness, multi-objective optimization, transparency, and user control. Rajput also touches on issues like hallucination, fabricating information, and the importance of diversity and compliance. He concludes by highlighting ongoing efforts in user education, monitoring, third-party audits, community involvement, and public input, stressing that fairness in recommendation systems is an ongoing, iterative process requiring continuous improvement and vigilance.
[publishpress_authors_box]