Part of the Deep Dive: AI Webinar Series
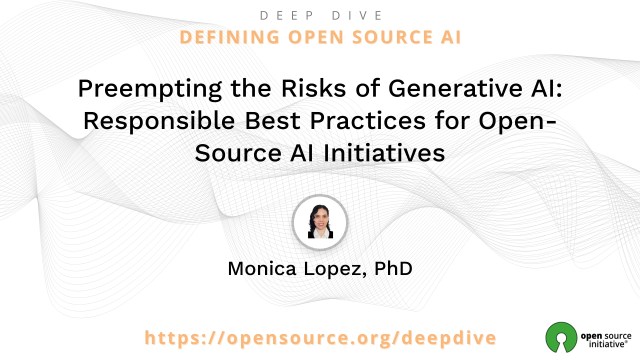
As artificial intelligence (AI) has proliferated across many industries and use cases, changing the way we work, interact and live with one another, AI-enabled technology poses two intersecting challenges to address: the influencing of our beliefs and the engendering of new means for nefarious intent. Such challenges resulting from human psychological tendencies can inform the type of governance needed to ensure safe and reliable generative AI development, particularly in the domain of open-source content.
The formation of human beliefs from a subset of available data from the environment is critical for survival. While beliefs can change with the introduction of new data, the context in which such data emerges and the way in which such data are communicated all matter. Our live dynamic interactions with each other underpin our exchange of information and development of beliefs. Generative AI models are not live systems, and their internal architecture is incapable of understanding the environment to evaluate information. Considering this system reality with the use of AI as a tool for malicious actors to commit crimes, deception –strategies humans use to manipulate others, withhold the truth, and create false impressions for personal gain– becomes an action further amplified by impersonal, automated means.
With the entrance in November 2022 of large language models (LLMs) and other multimodal AI generative systems for public use and consumption, we have the mass availability of tools capable of blurring the line between reality and fiction and of outputting disturbing and dangerous content. Moreover, open-source AI efforts, while laudable in their goal to create a democratized technology, speed up collaboration, fight AI bias, encourage transparency, and generate community norms and AI standards by standards bodies all to encourage fairness, have highlighted the dangers of model traceability and the complex nature of data and algorithm provenance (e.g., PoisonGPT, WormGPT). Further yet, regulation over the development and use of these generative systems remains incomplete and in draft form, e.g., the European Union AI Act, or as voluntary commitments of responsible governance, e.g., Voluntary Commitments by Leading United States’ AI Companies to Manage AI Risks.
The above calls for a reexamination and subsequent integration of human psychology, AI ethics, and AI risk management for the development of AI policy within the open-source AI space. We propose a three-tiered solution founded on a human-centered approach that advocates human well-being and enhancement of the human condition: (1) A clarification of human beliefs and the transference of expectations on machines as a mechanism for supporting deception with AI systems; (2) The use of (1) to re-evaluate ethical considerations as transparency, fairness, and accountability and their individual requirements for open-source code LLMs; and (3) A resulting set of technical recommendations that improve risk management protocols (i.e., independent audits with holistic evaluation strategies) to overcome both the problems of evaluation methods with LLMs and the rigidity and mutability of human beliefs.
The goal of this three-tiered solution is to preserve human control and fill the gap of current draft legislation and voluntary commitments, balancing the vulnerabilities of human evaluative judgement with the strengths of human technical innovation.
Webinar summary
In this webinar hosted by the Open Source Initiative as a part of the “Deep Dive: Defining Open Source AI” series, Dr. Monica Lopez, co-founder and CEO of Cognitive Insights for Artificial Intelligence, discusses the challenges posed by the proliferation of generative AI, particularly large language models (LLMs), focusing on two key issues: the influence of AI-generated content on human beliefs and the potential for nefarious uses of the technology. Dr. Lopez emphasizes that generative AI systems lack understanding and can produce biased, fabricated, and misleading content. She proposes psychological solutions, including toning down anthropomorphic language when describing AI capabilities and integrating empirical measurements of AI’s impact on human beliefs into system audits. Additionally, she addresses the risks of misuse, emphasizing the need for transparency, fairness, accountability, and reliability in open source AI initiatives. Dr. Lopez calls for benchmarks that align with societal goals and highlights the importance of interdisciplinary collaboration in addressing these challenges while placing human control at the center of AI development and use.
[publishpress_authors_box]